Adam Kinney, Head of Machine Learning at Mixpanel
04. April 2017
Take me to the German version of this article.
Mixpanel is one of the most popular data tools for large companies as well as for startups. In San Francisco, we spoke with Adam Kinney, Head of Machine Learning, and Amir Movafaghi, CEO, how they plan their roadmaps and how they see the future of data analysis and the role Mixpanel will play in it.
- In this text, you will learn:
- Why causal inference is the next big thing in ML.
- In which areas ML has the greatest potential.
- How Mixpanel aligns its product teams to the innovation loop.
- How Adam, as an engineering lead, does his roadmap planning.
- At what point you should start building your own data science team.
Adam Kinney feels most comfortable when he is not the smartest person in the room. At least, that’s what Adam says about himself when we met him for an interview at the headquarters of Mixpanel in San Francisco. There are two things behind this self-description. First, Mixpanel’s Head of Machine Learning and Analysis is obviously a very modest person. At the same time, Adam’s statement about himself reveals a second quality: his willingness to learn new things every day and openly discuss his own and everyone’s ideas and suggestions.
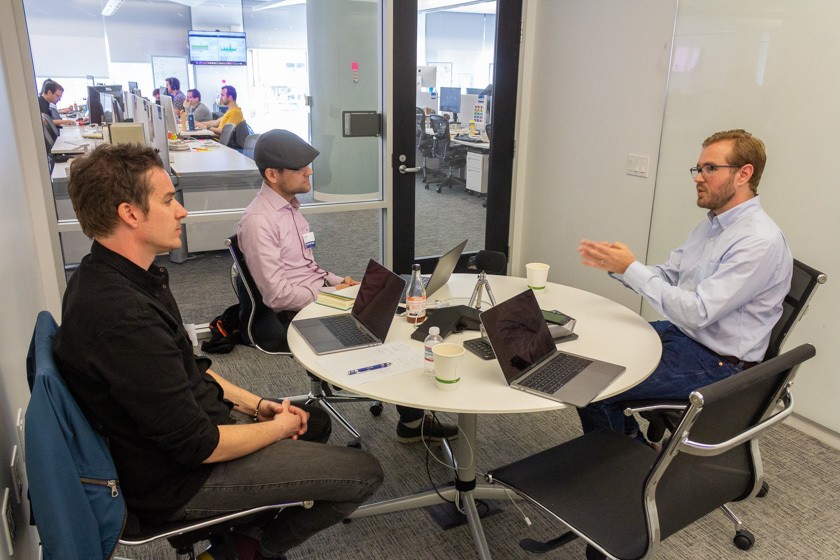
There is probably no area in which these skills are more useful than artificial intelligence and machine learning. Both science and business are just beginning to understand how this technology can be used to create real value for users. But on the horizon, what future versions of artificial intelligence will be capable of is already emerging. And in all modesty: Adam, who has already played crucial roles in statistical analysis at Google and Twitter, firmly believes that Mixpanel can have a say in this development. For him, this means constantly weighing up big, visionary ideas that take months to explore and develop against pragmatic use cases that can be implemented in two-week sprint cycles at Mixpanel.
About Adam
Asked for his leitmotif, Adam turns to the well-known quote about the computer as a „bicycle for our minds“ by Steve Jobs. Just as the computer has accelerated people’s work, Adam believes that machine learning will enable people to get things done even faster and more efficiently than before.
„We are still a long way from something like general intelligence.“
In this respect, Adam was particularly influenced during his time at Schibsted Media from 2014 to 2018, a large Norwegian media house that operates a number of classified ad portals, in addition to a number of daily newspapers.
At that time Adam was well on his way to a text-book career in Silicon Valley. After completing his degree in Statistics at Stanford he got his first job at Google in 2006. There he experienced how the tech giant slowly but surely became the leading authority on machine learning, Adam remembers. „I was one of the first people at Google who didn’t have a background as a developer but came out of statistical analysis. I was able to work with big data tools and technologies that most people hadn’t heard of yet.“
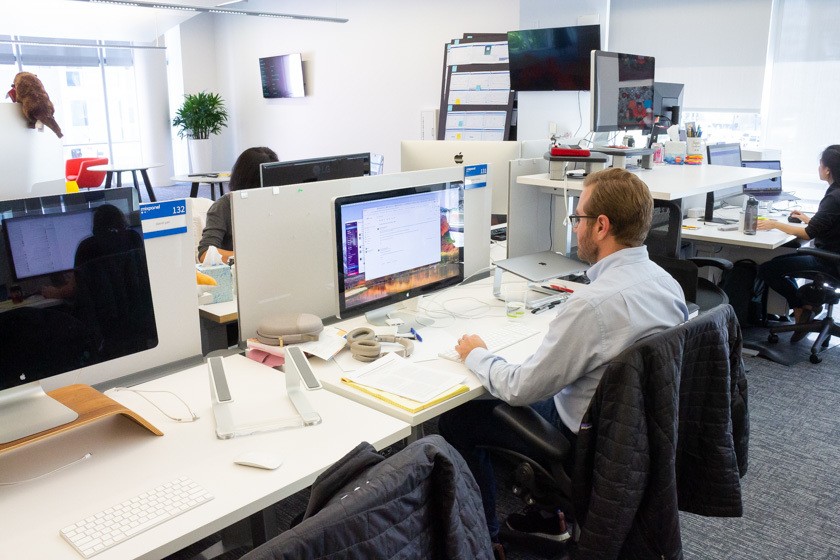
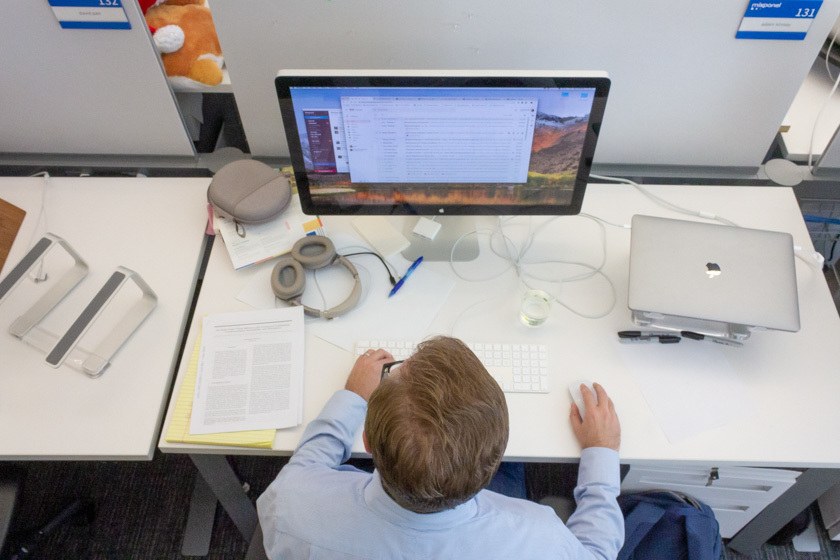
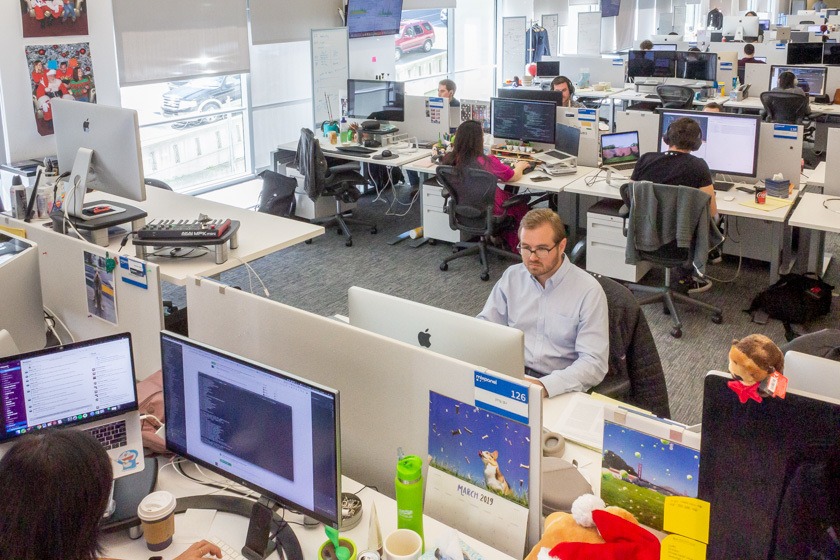
With this experience in mind, Adam moved to Twitter in 2010 to build an 18-person Data Science team. It was during this time that he first met Amir Movafaghi, now CEO of Mixpanel, who was responsible for Finance at Twitter. After another four years, and already having worked with data analysis for two of the most influential tech companies of our time, Adam would have had any door open to him in Silicon Valley. But he made a completely different decision: „I had never worked outside the Bay Area and wanted to see something new again.“ He decided to take the risk, accepted an offer from Schibsted and moved with his family across the Atlantic to Norway.
Machine Learning at a European media company
Adam got to know a different working culture and environment at the publishing house founded in 1839: „At Google, it’s the developers who run the show. That’s very impressive, but it’s also intimidating when you don’t have that kind of background.“ At Schibsted Media, Adam met just the editors and salespeople whose jobs have changed massively as a result of the products of his two former employers. The learning process started for the tech enthusiast, who had largely been influenced by the mindset of Silicon Valley: „In the beginning, I had the rather naive view of completely personalizing news pages and having them created by algorithms. Get rid of the editors and all that stuff! But when I saw how these people work and how editorial processes work, I realized where the real value would be,“ Adam remembers.
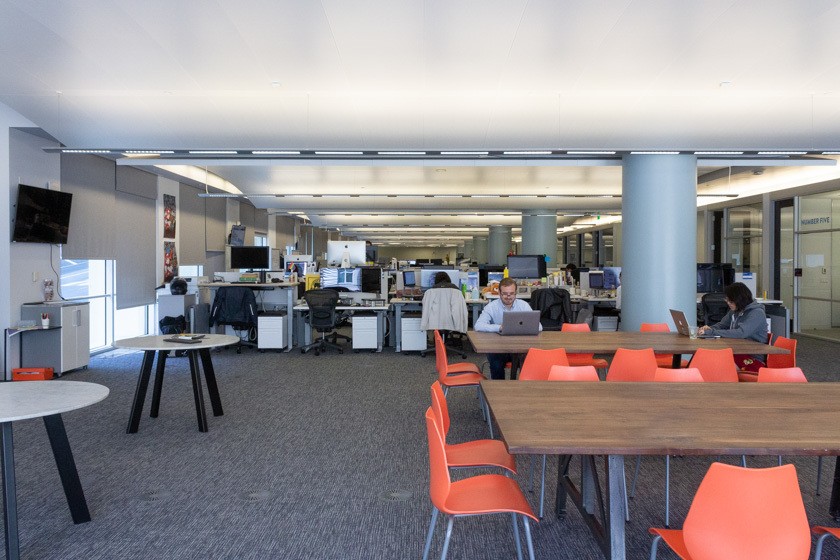
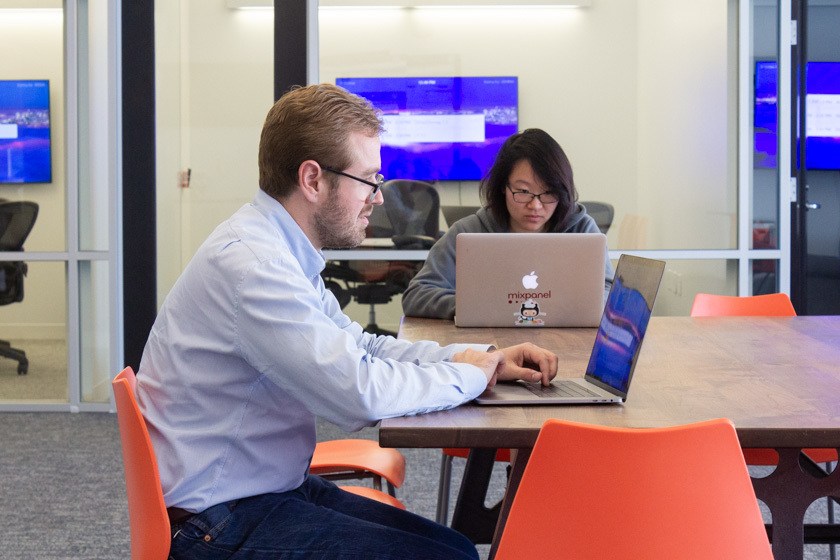
His focus shifted from replacing people’s work with technology to improving processes to make his colleagues‘ work easier. For Schibsted’s classifieds portals, he developed features that helped users create classified ads more easily and quickly through image recognition. He supported the editorial team with a tool that automatically assigned tags to articles using text recognition, so editors no longer had to do it themselves, thus freeing up resources.
Tech Jobs
- IT Expert / Requirements Engineer – Store Checkout Solutions (m/w/d) bei ALDI International Services SE & Co. oHG, Mülheim an der Ruhr
- Operations Expert – Store Checkout Solutions (m/w/d) bei ALDI International Services SE & Co. oHG, Mülheim an der Ruhr
- (Senior) Enterprise Architect (m/w/d) bei REWE Digital, Köln
- Data Engineer Schwerpunkt Kundenpersonalisierung (m/w/d) bei REWE Digital, Köln
- Software Engineer Fullstack (m/f/d) Angular .NET bei Verivox GmbH, Heidelberg
Adam’s European adventure with Schibsted Media ended in the summer of 2018. His former Twitter colleague Amir Movafaghi had just taken over the job of CEO at Mixpanel, a user behavioral analytics tool. When Adam congratulated him with a message, Amir promptly wrote back: „We should get together, we have some exciting things to do here.”
Living the Innovation Loop
Mixpanel, founded in 2010 by Suhail Doshi and Tim Tefren, had already defined the user behavior analytics sector but was planning to expand capabilities beyond identifying trends and surfacing metrics. The plan was to incorporate the so-called Innovation Loop. As explained by CEO, Amir Movafaghi: „This loop is not an invention of ours. The cycle has been followed by innovative companies for many years. But it now forms the pillars of our product development.“ The five steps are as follows.
- Ingestion: The first step is to collect accurate data from all of the different channels and platforms.
- Identify: Graphs and diagrams bring the data into formats that can be understood and processed by the teams.
- Analytics: Now you can read the data and try to recognize correlations and patterns between the individual data points.
- Interpretation: The next step is to interpret these relationships correctly and to understand why the data is what it is. To do this, hypotheses are formed and tested.
- Take Action: This results in concrete options for actions. You want to achieve a certain change in the user’s behavior, for example, „More newsletter subscriptions“. Based on the data, you know which screws to tighten. This results in new data points and everything comes full circle.
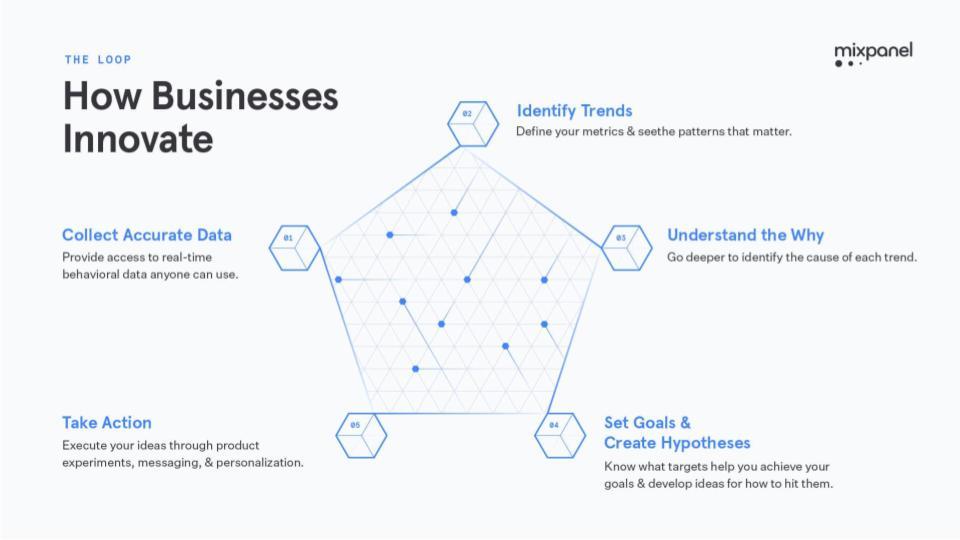
The aim of Mixpanel is to accelerate this process as much as possible. Each team is assigned to one of these pillars, explains Amir: „We want to put companies in a position to test ideas efficiently so that they can quickly find out what works and what doesn’t. Those who can do this have an immense market and competitive advantage to be agile and disruptive. That’s how we’ve seen companies like Slack and Airbnb quickly find product-market fit, test new ideas, and disrupt giants. And it’s the advantage that will help larger companies continue to innovate and invest their immense resources in the right areas.“
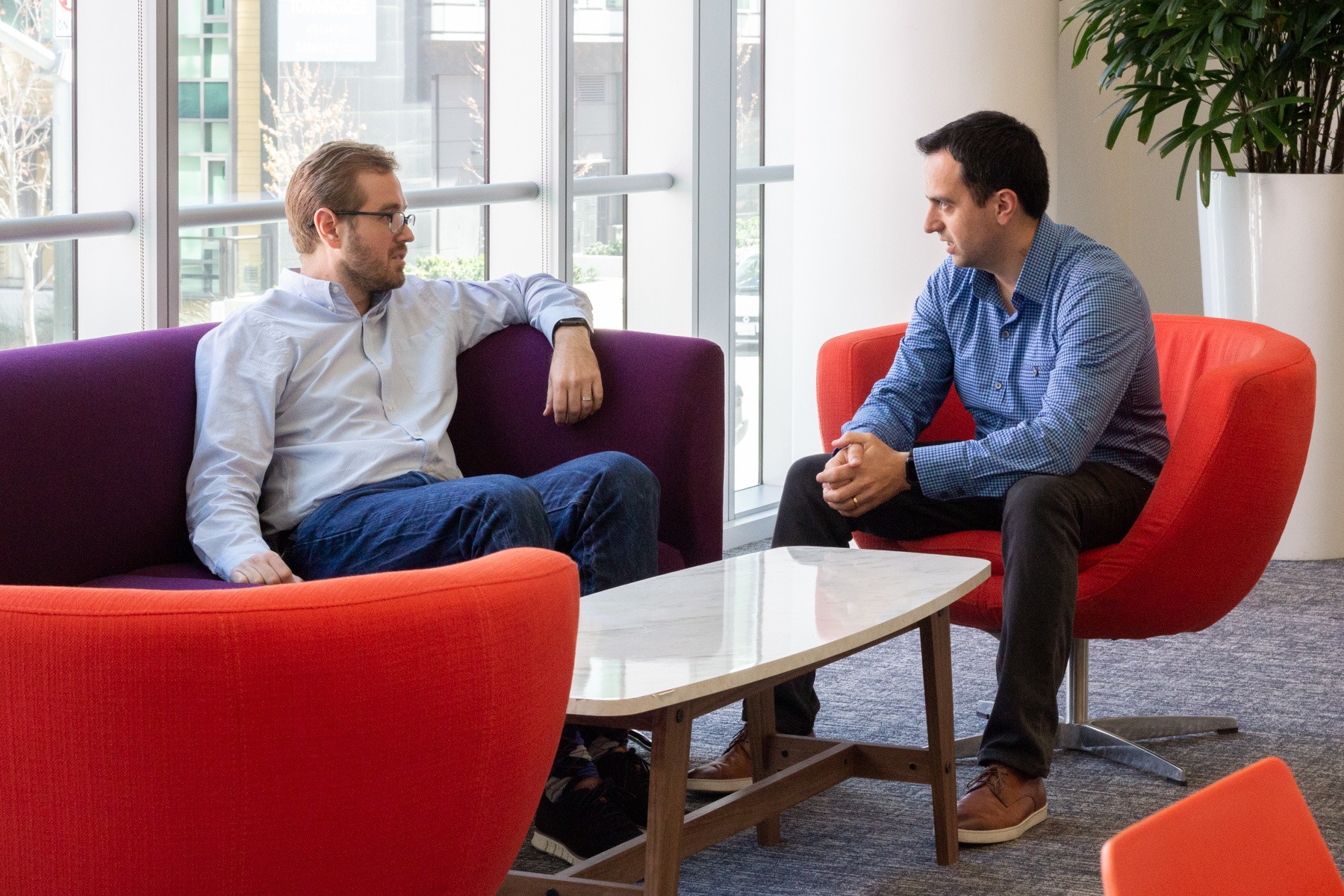
As Head of Machine Learning and Analysis, Adam and his team are particularly involved in steps three and four, analysis and interpretation. Machine learning at Mixpanel is basically always about data analysis, but not everything in the analysis is automatically machine learning, so Adam works closely with his colleagues from the analysis team.
A few features of the Machine Learning team are stand-alone, such as „Anomaly Detection,“ Adam explains. If you have an unusual spike in a metric, you automatically get a notification of this behavior to allow you to quickly take action. Another feature is called „Predict,“ which forecasts how likely users are to perform an event and assigns a confidence. This allows triggering certain actions upfront, such as sending a discount code before a product is purchased.
Short- and Longterm Road-Maps
Adam’s thoughts revolve almost around the clock on the possibilities and limitations of machine learning and how to use the technology for Mixpanel’s products. He collects his ideas in various Google Docs („And I have a whole bunch of them.“) and works them out further. He gives the smaller, quickly implementable initiatives directly to the teams.
It’s important to Adam that his ideas are discussed and challenged just like everyone else’s: „If I’m the first to present my idea about a problem, everybody tends to anchor around it. That’s why we meet in a big room where everyone can come up with three ideas. The wilder, the better. We collect all suggestions on sticky notes and cluster them in a spreadsheet. Then everyone votes on a scale from 1 to 5, which would bring the greatest value to our customers. Some of my ideas get through, but often they’re dismissed, which I think is great.“
This process helps the team develop a rough prioritization. The concrete roadmap is defined by the teams themselves. The engineering leads set the guidelines and make sure that the teams don’t get bogged down, Adam says: „We’re not very process-heavy. It’s important to me that there’s a clear direction for the projects with the highest priority. I’m always ready to change course when we get new user data or other information. But it’s important to keep the focus and rather do a few things very well than many things mediocre.“
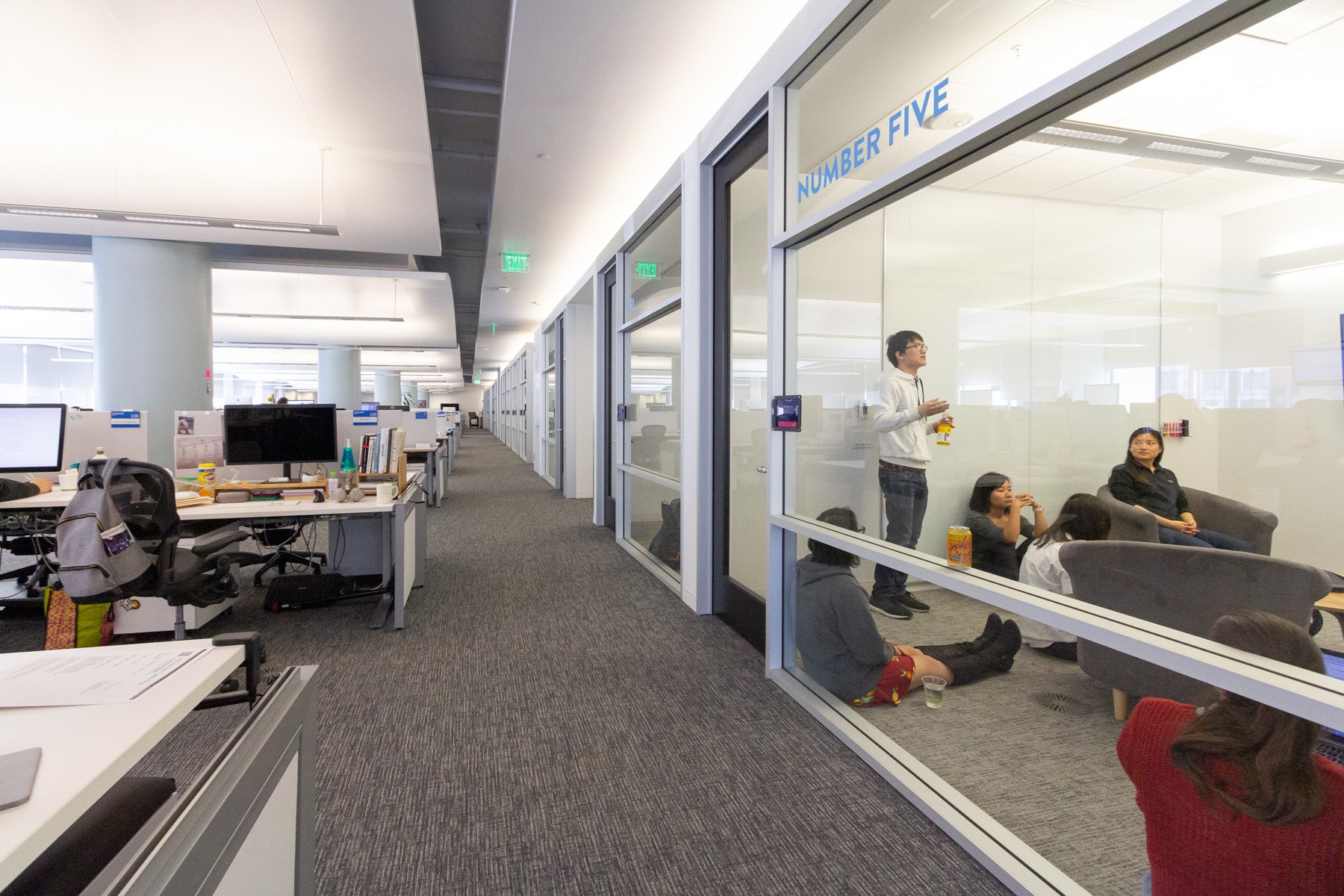
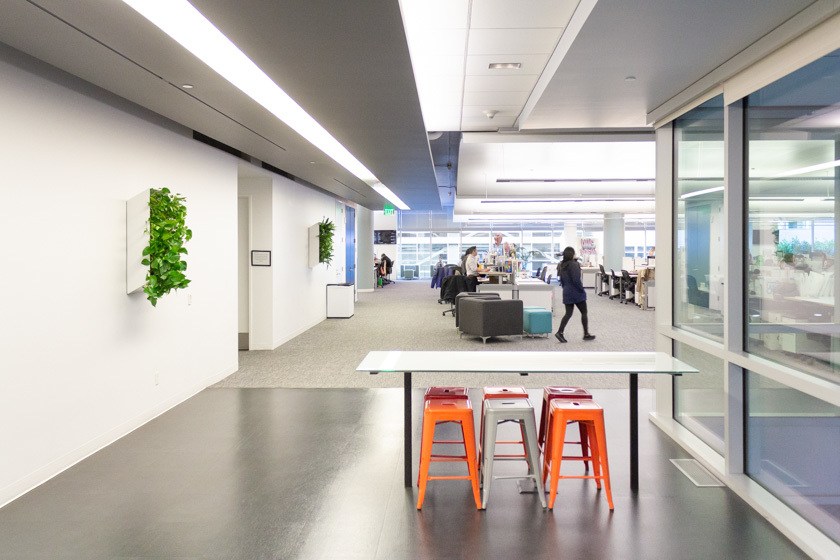
Adam first shares the larger initiatives with the other engineering leads and the respective product managers and gets feedback. „This is a process that can take weeks. Before the first line of code is written, I talk to the product manager until we are completely on the same page about what such a project can look like.” An example of such a big initiative is trying to solve one of the fundamental problems of statistical analysis: Uncovering causal relationships between two or more variables.
Causal Inference: The Next Big Thing
The core problem is that a machine can quickly evaluate the data but to successfully interpret this data and draw the right conclusions they need very advanced algorithms. User behavior is complex. Why do some people buy something in a shop and others don’t? Why do some users stop an onboarding process at one point while others proceed smoothly? Why does the usage time in the app go down after a new feature got released? To truly understand these questions, you need not only the data but also the empathic understanding of human minds. In product development these days, decisions are mainly made on the basis of user data. One risk here is to confuse a correlation with causation, thus gathering misleading insights and making wrong product decisions.
Adam wants to reduce this risk with Mixpanel, he explains: „I experienced this myself in my time as a data scientist. When I looked at the data, I thought I had found an explanation for a particular phenomenon. But when we did an A/B test, there was nothing there. That’s very frustrating.“ The difficulty is in controlling the confounding variables.
A well-known example of this phenomenon is that people with larger shoe sizes have higher income. However, it’s not big feet that inevitably lead to a dream salary. The truth behind these statistics is different: Men tend to be better paid than women and often have larger feet for biological reasons. As soon as the statistics are broken down by gender, the correlation disappears. This statistic can be easily resolved because the third variable „gender“ is clearly defined. But what about complex characteristics such as user behavior?
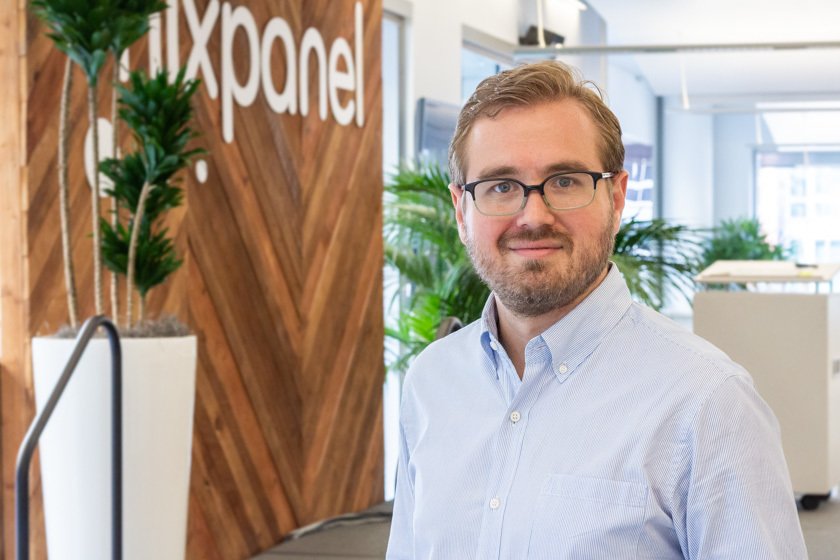
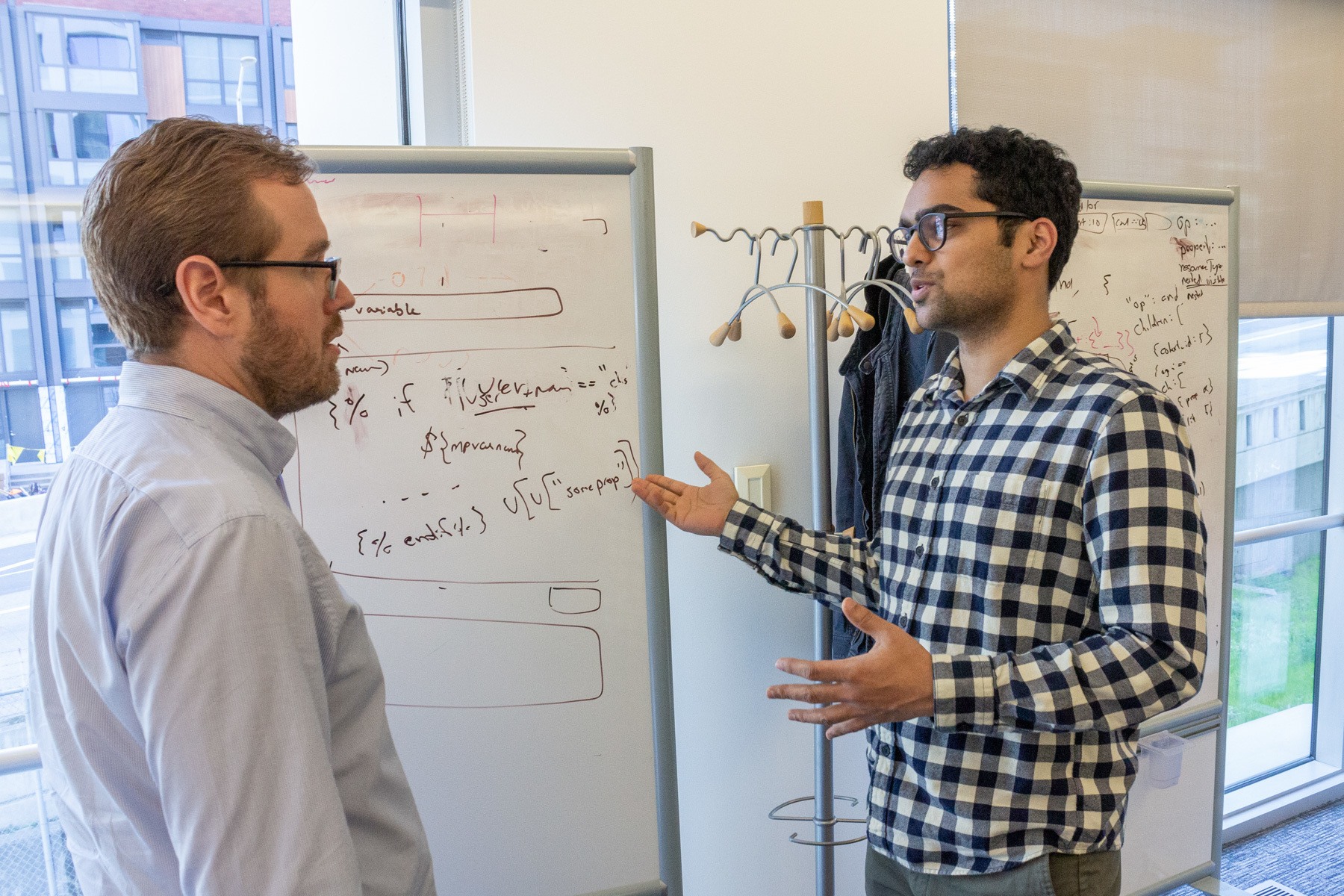
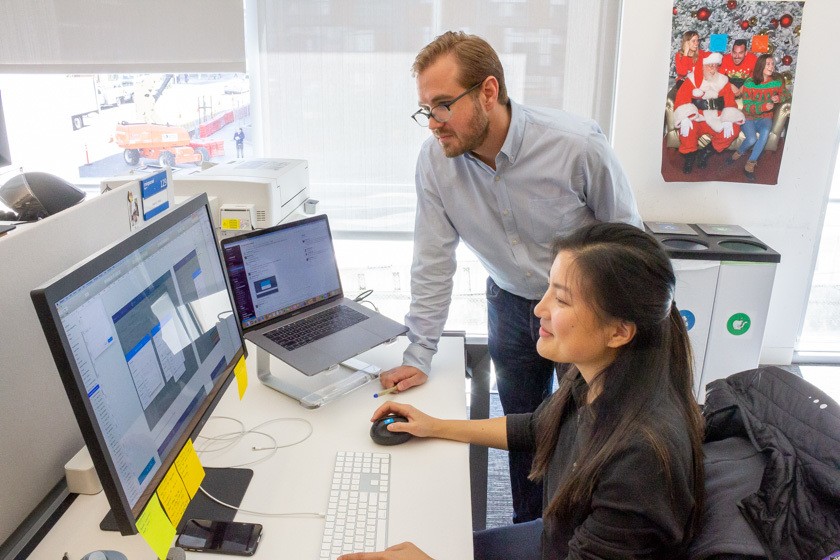
Adam gives a (constructed) example from e-commerce: People who frequently write product reviews buy more online than people who do not write reviews. If this is true and there is a causal relationship, it would make sense to encourage more reviews to increase revenue. But it could also be that there is a group of hardcore users who simply find online shopping incredibly good and are more engaged on all levels than other users. That would explain why there is a correlation between willingness to buy and writing reviews, but no causal relationship. In this case, it would probably be more impactful to try to drive customer loyalty and turn more light or average users into hardcore users. Depending on which explanation is correct, different features have to be developed and different user groups get addressed.
But in order to find out which way is right, the shop provider would actually have to spend a lot of resources and effort over a long period of time. Valid criteria for hardcore users is needed to distinguish them from other users. Developers need to build a working feature that encourages users to write more reviews. Then the product team would have to monitor over a period of time which user group’s reviews actually lead to more sales and check the information against the control groups in order not to fall for a fictitious correlation again.
„You can spend half a year just doing that and then find out that you were wrong.“
And this example does not yet take into account the fact that in reality there could be not just one, but ten possible variables that cause this effect. If you don’t have a large data science team and corresponding resources in development, you can’t do such analyses.
Tools like Mixpanel can facilitate this process, Adam believes. „Causal inference is the next big thing in machine learning. The idea is to use statistical methods to create a model that predicts the most likely explanations for particular user behavior. Of course, you will never end up with 100 percent accuracy but if you’re able to isolate the three most likely out of ten possible causes, a lot would be gained,“ Adam explains. This would simplify decision-making processes and help prioritize product teams. It would also allow companies to better allocate their data analysis resources. “Building a data science team is not a trivial thing,” Adam says.
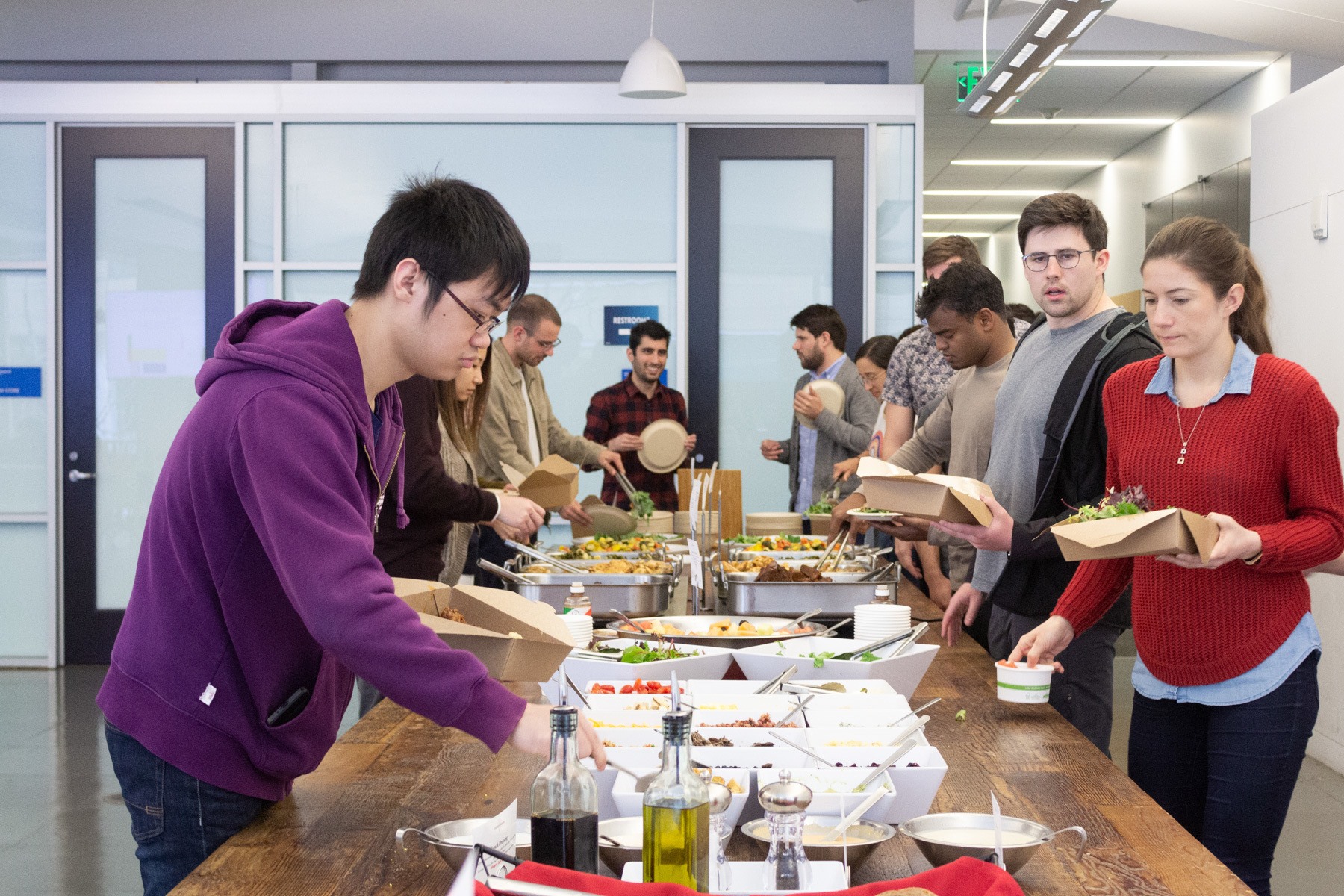
Why you shouldn’t hire too early
„I have worked in this area myself and understand a lot of the problems and frustrations in this job. I believe that many companies start hiring their own data scientists too early. For these experts to really solve problems, they need data infrastructure with high quality data. Especially in smaller and early-stage companies, the company as a whole is not there yet. This means that a data scientist can identify problems and explain how to solve them but the developers don’t have the resources to actually do so. This creates frustration and a feeling of powerlessness.“
It is much more important to collect the data cleanly from the start and log it in a data warehouse. „A good backend developer can do this on his own by stitching together a few cloud services.“ Only when there is no one left in the company who can answer certain questions about the data with onboard resources does it become time to hire someone new.
For Adam, it’s a question of prioritization: „If you have the choice to hire a full-time data scientist, or invest in the existing development team, and have them build a simple analytics setup, it’s probably more valuable doing the latter.“
But Adam also has some advice for upcoming data scientists: They should be able to talk to the developers in their language.
„I took more programming classes than I had to during my studies, and that was very important to me.”
Coming back full circle to Adams‘ initial statement of not wanting to be the smartest room- this probably means another thing. If you really want to become successful as a data scientist, you’ll need to make sure you’re not easily outsmarted by the data.
The interviews and photo shootings for this article took place in Mixpanel’s former office. Meanwhile, they have moved to a new space. You can read all Digitale Leute articles that have been developed in San Francisco here.
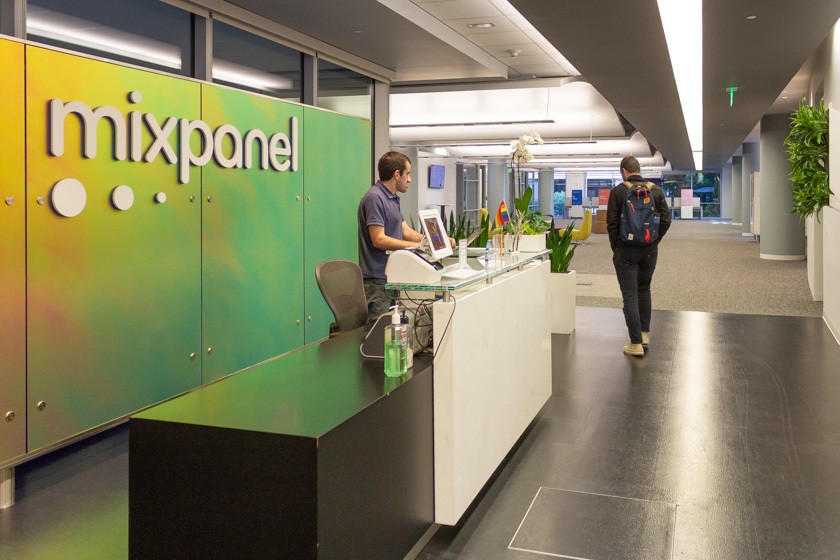